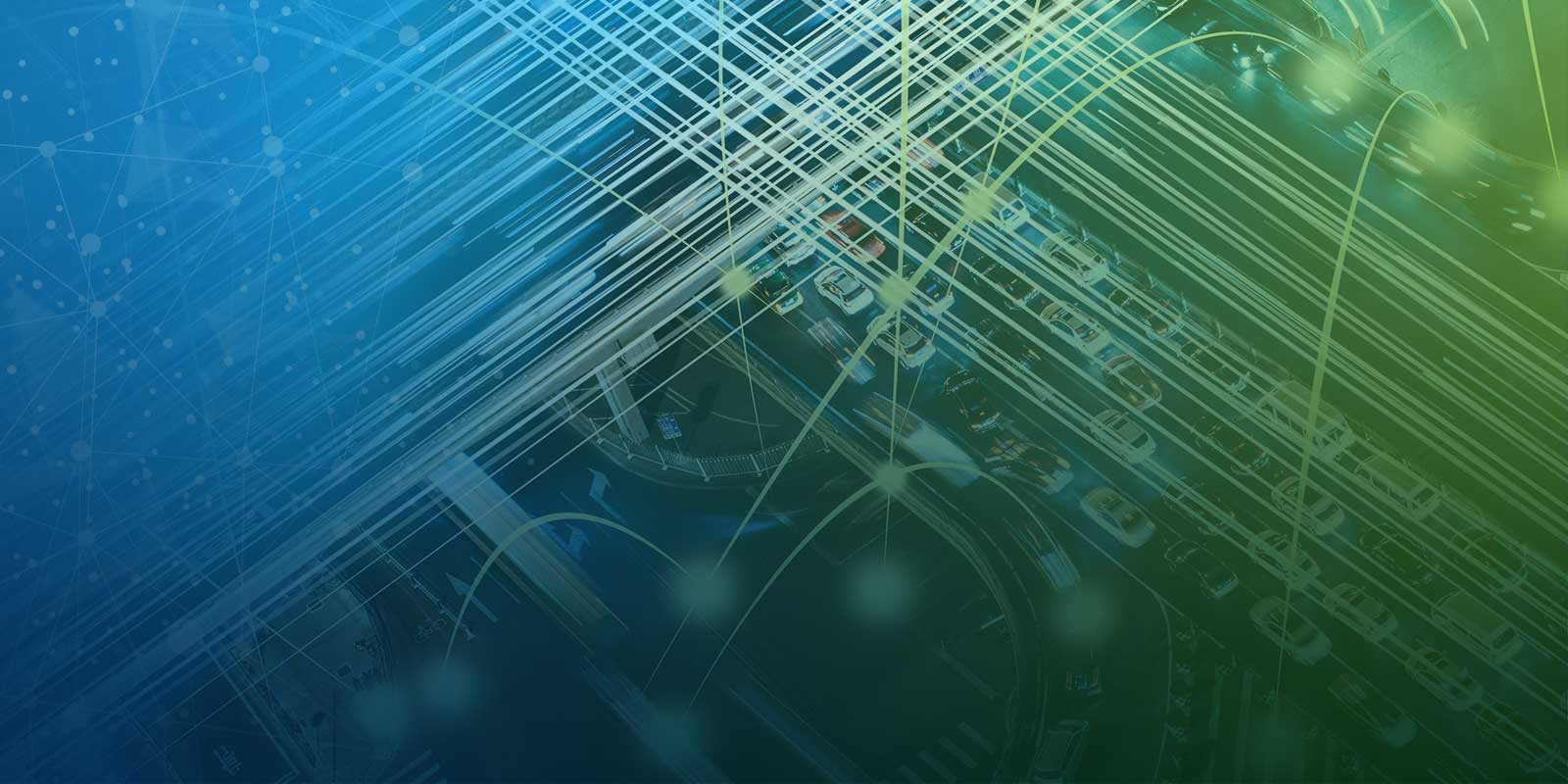
Over the past decade, the US has seen profound changes in how many of us interact with the transportation systems. For instance, in 2010, we had only just started using Uber/Lyft and had not fully realized the potential of their offerings. Bike-share systems were just gaining a foothold in the major metro areas across the US and Amazon Prime had not yet become (in)famous for increasing the strain on our transportation infrastructure with free one-day deliveries. Mobility-as-a-service, connected and autonomous vehicles (CAV), and electric vertical takeoff and landing (eVTOL) were all just concepts whispered about in the hallways of industry conferences and meetings.
Many of these innovations were made possible by the rapid advances in the design and development of smartphones and their ability to connect to reliable internet. This mobile-based internet connection has also changed the way we spend our time as we walk, run, take transit, drive, or bicycle. Who amongst us hasn’t heard a gripping podcast as we take the train or used a navigation app for directions while simultaneously attending a Zoom call?
How has the private sector used smartphone data to inform decisions?
The increase in smartphone use and applications have created a secondary industry--the production and utilization of location and timestamp data. The basic premise is this: using location information generated from the apps, advertisers and content managers are able to generate highly curated and customized content (e.g. localized news) and serve tailored ads (e.g. discounts at the local clothing retailer). When packaged in the right format these powerful data have also helped make commercial real-estate decisions, predict future stock movements, and support banking and credit analytics.
What about the transportation industry?
The world of transportation planning and modeling that I work in also benefited tremendously from this multifold increase in locational inference data. For decades, travel surveys and traffic counters were the only reliable ways to understand travel behavior and build predictive traffic models. And, because surveys are expensive to conduct, public sector agencies only conducted a limited set of surveys and only about once in a decade. The new location data upended the prevailing norms of information gathering with large sample sizes and ongoing data refreshes. Innovative companies that saw a gap in these data collection events harnessed this information and brought the data-as-a-service model to the transportation planning industry. The adoption rate for using these data, in what has historically been a thoughtful and cautious industry, was rapid. Within a year or two, data were being used for all kinds of use-cases: before and after assessments, update of transportation models, and to study special event (e.g. concerts) movements.
There have been two main reasons for caution in using these data in the transportation planning realm. They are:
To support high-value investments, transportation planning and models need to answer five questions about travel: when, where, who, why, and how. Location data do a fantastic job answering the when and where questions. With good thought and research, the why has been answered satisfactorily as well. But, the who (is making the trip) and how (on what mode) questions are much harder to solve using these data without impeding on user privacy.
While the sample sizes are large, these data are only a sample. Without accounting for biases and performing rigorous expansion, the data could lead to assigning higher priority to transportation needs of those using smartphones frequently and often, making it a challenge to look at the full breadth of population through an equity lens.
In 2018, in partnership with LA Metro and Caltrans, my colleagues and I at Cambridge Systematics (CS) developed a beta version of an analytical product that married the strength of the location data with insights that we at CS have learnt from our 45 years of work in travel behavior and transportation modeling. This work led to the development of LOCUS, our suite of location-based data products.
The core tenets of LOCUS are:
Always use the product to build an equitable and just transportation future – this means acknowledging and addressing (to the extent that we can) the biases that are intrinsic to the data.
Value the privacy of the users that generate the data streams. So, in all our projects, we have prioritized privacy over spatial and temporal granularity;
Customize and validate delivery to local conditions and specific project needs by blending LOCUS data with other transportation survey and behavioral information;
Be honest about the limitations of the data and our product right from the start. We want to align with partners who will use our product the right way; and
Mold our solutions to support existing tools that transportation agencies already have. Many of our clients operate on tight budgets, we don’t want to burden them with yet another “tool” that cannot integrate with the models, plans, or solutions that they are already using.
Since delivering our first two projects, LOCUS has powered models, plans, and operations for an additional 30 transportation agencies across the U.S. LOCUS has been used for redesigning bus systems in major metro areas such as Los Angeles, Seattle, Dallas, San Diego, Denver and Boston; studying climate events in Florida and Southern California; updating transportation models in California and New York; studying the impacts of COVID-19 on transportation needs and transit recovery; and helping speed/reliability/curb enhancements. And, all along the way, we have learnt from our partners and modified our solutions to meet their needs.
Over the next year, we will build out our product even more, always in consultation with our agency partners and always keeping our core tenets in mind.
Reach out to us at akomanduri@camsys.com and pvinayak@camsys.com if you are interested in learning more about LOCUS.